Huawei's Ascend 910B: Design Differences Exposed
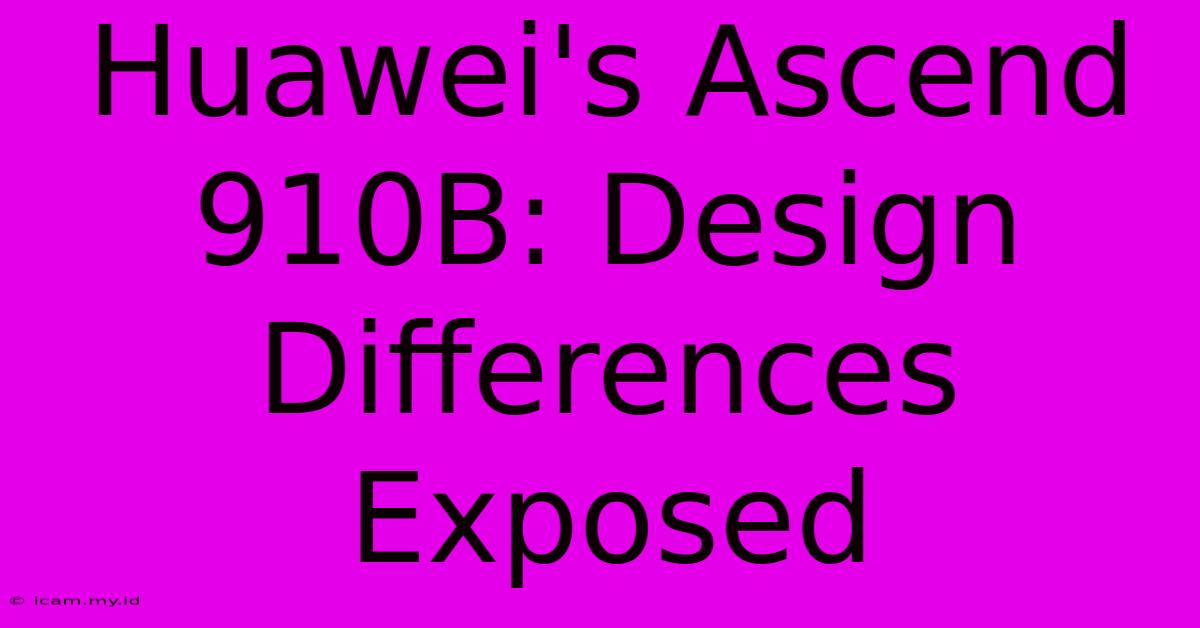
Find more detailed and interesting information on our website. Click the link below to start advanced information: Visit Best Website meltwatermedia.ca. Jangan lewatkan!
Table of Contents
Huawei's Ascend 910B: Design Differences Exposed
The Ascend 910B, a high-performance AI training processor from Huawei, has garnered significant attention within the tech community. While its impressive specifications are widely publicized, the intricate design differences that set it apart from competitors remain less explored. This article delves deep into the architectural nuances of the Ascend 910B, comparing it to other leading AI processors and exposing the key design choices that contribute to its unique capabilities.
Understanding the Ascend 910B's Architecture
At its core, the Ascend 910B is a massively parallel processing unit (MPU) optimized for deep learning training. Unlike general-purpose CPUs or GPUs, the Ascend 910B's architecture is meticulously tailored for the specific demands of AI workloads. This specialized design allows for unparalleled performance and efficiency in training large, complex neural networks. Key architectural features include:
-
High-Bandwidth Interconnect: The Ascend 910B boasts a high-bandwidth interconnect, enabling seamless communication between its numerous processing cores. This high-speed communication is crucial for efficient data transfer during training, significantly accelerating the overall process. The design minimizes bottlenecks often encountered in other architectures, allowing for near-linear scaling with the number of cores.
-
Custom Instruction Set Architecture (ISA): Huawei designed a custom ISA specifically for AI workloads. This tailored instruction set optimizes for the specific operations frequently used in deep learning, such as matrix multiplication and convolution. This approach, unlike relying on general-purpose ISAs, minimizes instruction overhead and maximizes computational efficiency.
-
Massive Parallel Processing: The Ascend 910B’s architecture prioritizes massive parallelism. This involves distributing the computational workload across a vast number of cores, enabling it to handle the immense computational demands of training large-scale AI models. The parallel processing capability allows for significant speedups compared to systems with fewer, more powerful cores.
-
High Memory Bandwidth: Training large AI models requires substantial memory bandwidth to keep the processing cores supplied with data. The Ascend 910B addresses this need with a high-memory bandwidth design, ensuring sufficient data flow to prevent performance bottlenecks. This design choice minimizes data transfer latency and maximizes throughput.
Comparing Ascend 910B to Competitors: Unveiling the Differences
To fully appreciate the Ascend 910B's design ingenuity, comparing it to prominent competitors such as NVIDIA's A100 and Google's TPU v4 is essential. While all three are high-performance AI training processors, their architectures differ significantly, leading to varying strengths and weaknesses:
Ascend 910B vs. NVIDIA A100:
The NVIDIA A100, a flagship GPU, relies on a more general-purpose architecture compared to the Ascend 910B's specialized design. While the A100 offers excellent performance across a broader range of workloads, the Ascend 910B excels specifically in deep learning training due to its custom ISA and optimized interconnect. The A100 might offer better support for mixed-precision computations in some scenarios, but the Ascend 910B's focus on training large models provides a competitive edge in certain applications. The key difference lies in the targeted application: the A100 is more versatile, while the Ascend 910B is highly specialized for AI training.
Ascend 910B vs. Google TPU v4:
Google's TPU v4, like the Ascend 910B, is highly specialized for AI training. However, Google's approach differs in the interconnect strategy and memory management. TPU v4 excels in certain aspects, particularly in inter-chip communication within a larger system. The Ascend 910B, on the other hand, might offer advantages in terms of power efficiency for comparable performance levels. The comparison boils down to a subtle difference in architectural optimizations, resulting in nuanced performance variations depending on the specific AI model and training configuration. Both are leading processors, but their strengths are manifested in distinct application areas.
The Impact of Design Choices on Performance and Efficiency
The design differences between the Ascend 910B and its competitors directly impact performance and power efficiency. Huawei's focus on a custom ISA, high-bandwidth interconnect, and massive parallelism translates to significant speedups in training large-scale AI models. This specialized approach, however, might limit its applicability to other workloads not directly related to AI training.
The Ascend 910B’s optimized architecture also contributes to improved power efficiency. By minimizing unnecessary computations and optimizing data transfer, the processor reduces energy consumption compared to less specialized alternatives. This efficiency is critical for large-scale deployments where energy costs are a significant factor.
Future Implications and Potential Advancements
The Ascend 910B represents a significant milestone in AI processor design. Its specialized architecture and focus on massive parallelism pave the way for faster and more efficient training of increasingly complex AI models. Future advancements in this area may focus on:
-
Further Increases in Parallelism: Future iterations could incorporate an even larger number of processing cores, enabling the training of even larger and more complex models.
-
Improved Interconnect Technology: Advances in interconnect technology could lead to even higher bandwidth and lower latency, further accelerating training speeds.
-
Enhanced Memory Management: Optimizations in memory management could further improve efficiency and allow for the training of models requiring even greater memory capacity.
-
Integration with other Huawei Technologies: Integration with other Huawei technologies such as their cloud infrastructure could significantly expand the potential applications of the Ascend 910B.
Conclusion: A Specialized Powerhouse
The Ascend 910B's architecture stands out due to its specialization for AI training. Its custom ISA, high-bandwidth interconnect, and massive parallelism offer significant advantages over more general-purpose alternatives in specific applications. While comparisons with competitors like the NVIDIA A100 and Google TPU v4 reveal nuanced performance differences depending on the specific workload, the Ascend 910B's strengths lie in its efficiency and prowess in training large, complex deep learning models. The future iterations of this technology promise even greater advancements in the field of artificial intelligence. The exposed design differences highlight Huawei's strategic focus on creating a specialized, high-performance processor tailored for the ever-growing demands of AI. This approach, while potentially limiting its versatility, allows for exceptional performance in its targeted domain.
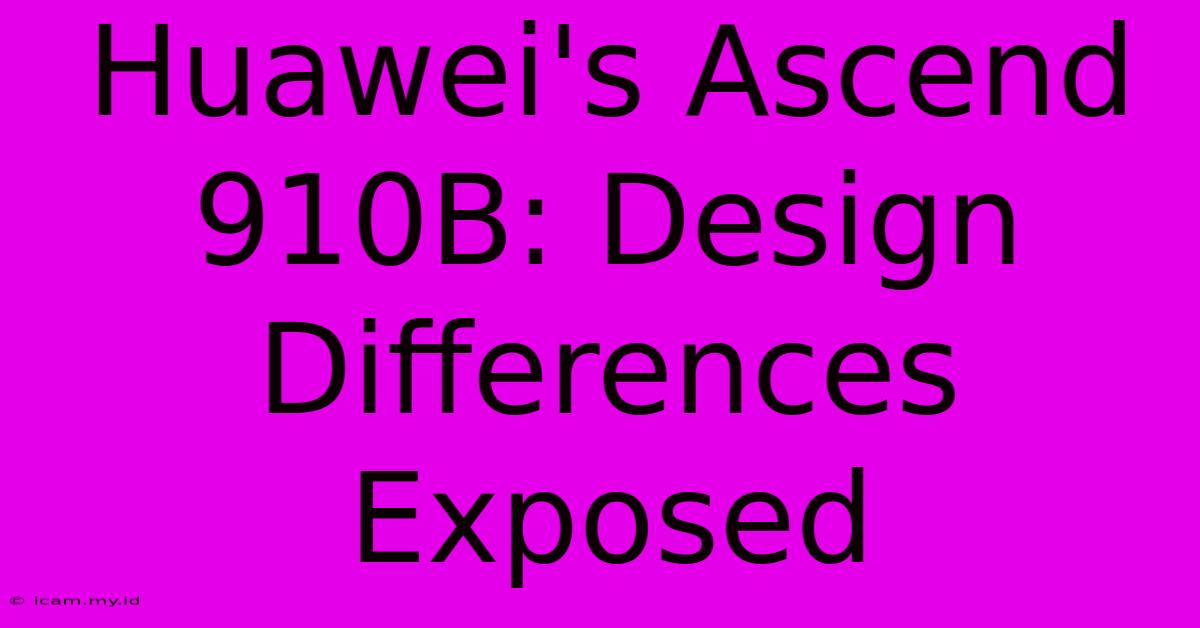
Thank you for visiting our website. Huawei's Ascend 910B: Design Differences Exposed. We hope the information we provide is helpful to you. Feel free to contact us if you have any questions or need additional assistance. See you next time, and don't forget to save this page!
Kami berterima kasih atas kunjungan Anda untuk melihat lebih jauh. Huawei's Ascend 910B: Design Differences Exposed. Informasikan kepada kami jika Anda memerlukan bantuan tambahan. Tandai situs ini dan pastikan untuk kembali lagi segera!
Featured Posts
-
Recap Colorado Dominates Oklahoma State
Nov 30, 2024
-
10 000 Idr Nutrition Program
Nov 30, 2024
-
Sanders Sets New Colorado Football Mark
Nov 30, 2024
-
Analyzing The Semiconductor Packaging Market
Nov 30, 2024
-
Airbus A330neo A New Era For Malaysia Airlines
Nov 30, 2024