Recent Empirical Report: Bioinformatics Publication
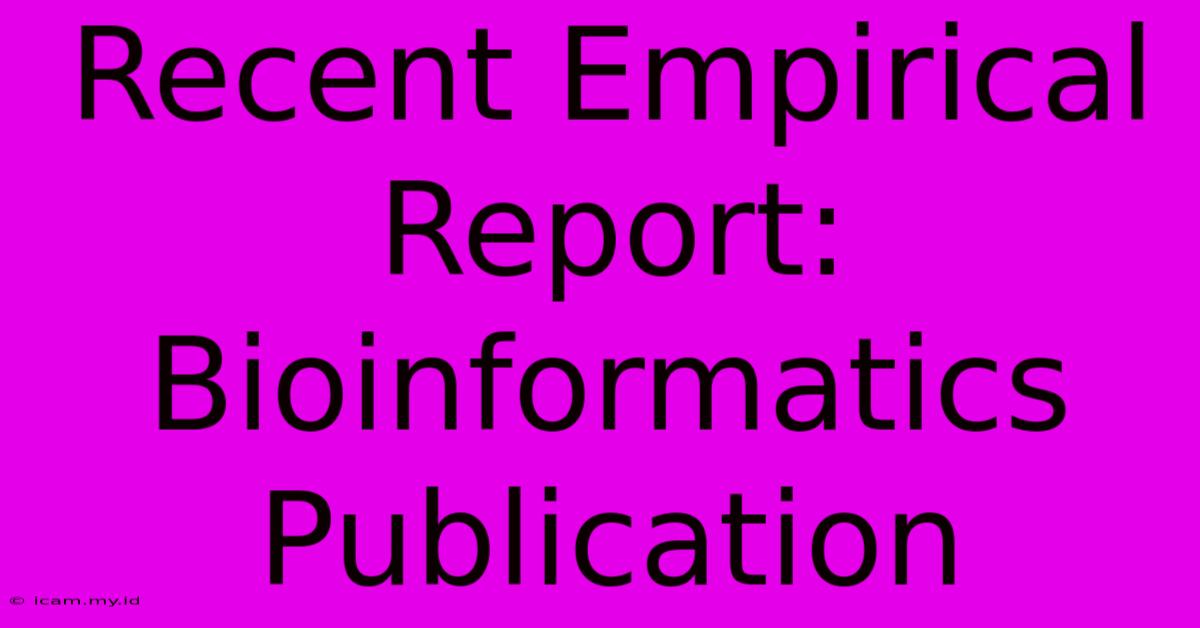
Find more detailed and interesting information on our website. Click the link below to start advanced information: Visit Best Website meltwatermedia.ca. Jangan lewatkan!
Table of Contents
Recent Empirical Report: Bioinformatics Publication
The field of bioinformatics is exploding, with a constant influx of new data and sophisticated analytical techniques. This necessitates a critical examination of the recent empirical reports published within this dynamic area. This article delves into the current landscape of bioinformatics publications, focusing on trends, challenges, and the future direction of the field as reflected in recent empirical studies.
The Rise of Big Data in Bioinformatics Publications
Recent years have witnessed an unprecedented surge in biological data generation, fueled by advancements in high-throughput sequencing technologies, microarrays, and other ‘omics’ approaches. This has directly impacted the nature of bioinformatics publications. Many recent empirical reports focus on the development and application of novel algorithms and statistical methods designed to handle and analyze these massive datasets. These publications often involve:
-
Next-Generation Sequencing (NGS) data analysis: A large proportion of recent bioinformatics papers address challenges associated with NGS data, including read mapping, variant calling, genome assembly, and transcriptome analysis. Empirical studies often compare the performance of different algorithms and software tools on various datasets, highlighting their strengths and limitations.
-
High-dimensional data analysis: ‘Omics’ technologies generate high-dimensional data, demanding sophisticated statistical methods to identify patterns and relationships. Recent empirical reports frequently explore novel dimensionality reduction techniques, machine learning algorithms (like support vector machines, random forests, and neural networks), and network analysis approaches to extract meaningful biological insights from this complexity.
-
Integration of multi-omics data: An increasing number of studies aim to integrate data from different ‘omics’ platforms (genomics, transcriptomics, proteomics, metabolomics) to achieve a more holistic understanding of biological systems. These empirical reports often focus on the development of integrative analysis methods and the biological implications of integrating diverse data types.
Challenges in Analyzing Big Data
Despite the advancements, analyzing big data in bioinformatics presents significant challenges, frequently reflected in recent empirical publications:
-
Computational resources: Analyzing massive datasets requires substantial computational power and storage capacity, often limiting the scope and scale of analyses. Many recent empirical reports discuss strategies for optimizing computational efficiency, including parallel computing and cloud-based solutions.
-
Data heterogeneity and standardization: Data generated from different sources and platforms can be highly heterogeneous, making integration and analysis challenging. Empirical studies often investigate methods for standardizing data formats and harmonizing data from diverse sources.
-
Data interpretation and visualization: Extracting meaningful biological insights from complex data requires sophisticated data visualization techniques and careful interpretation of results. Recent empirical reports often focus on the development of innovative visualization methods and strategies for validating bioinformatics predictions.
The Impact of Artificial Intelligence (AI) on Bioinformatics Publications
Artificial intelligence and machine learning are rapidly transforming bioinformatics. Recent empirical reports increasingly showcase the application of AI in various areas, including:
-
Drug discovery and development: AI algorithms are used to predict drug targets, design novel drugs, and personalize treatments. Empirical studies evaluate the performance and accuracy of these AI-powered approaches.
-
Disease prediction and diagnosis: Machine learning models are trained on large datasets of patient information to predict disease risk and diagnose illnesses. Recent empirical reports assess the effectiveness of these models in different clinical settings.
-
Genomic prediction and personalized medicine: AI is used to predict an individual's response to different treatments based on their genetic makeup. Empirical studies investigate the accuracy and clinical utility of genomic prediction models.
Ethical Considerations and Reproducibility in AI-driven Bioinformatics
The widespread use of AI in bioinformatics raises crucial ethical considerations, often discussed in recent publications:
-
Bias in algorithms: AI algorithms can inherit biases present in the training data, leading to unfair or discriminatory outcomes. Recent empirical reports highlight the importance of addressing bias in algorithms and ensuring fairness in AI-driven applications.
-
Data privacy and security: The use of sensitive patient data in AI-driven bioinformatics necessitates robust data privacy and security measures. Recent empirical publications emphasize the need for ethical guidelines and regulatory frameworks to protect patient data.
-
Reproducibility of results: Ensuring the reproducibility of AI-driven bioinformatics analyses is crucial for validating findings and building trust in the field. Recent empirical reports discuss strategies for improving reproducibility, such as sharing code and data, and using standardized methods.
Future Directions in Bioinformatics Publications
The future of bioinformatics publications will be shaped by several key trends:
-
Increased integration of experimental and computational approaches: Future studies will likely emphasize the synergistic integration of experimental data with computational analyses to achieve a more comprehensive understanding of biological systems.
-
Development of more sophisticated and robust algorithms: The demand for more efficient and accurate algorithms to analyze increasingly complex datasets will drive further innovation in algorithm design and optimization.
-
Focus on translating bioinformatics research into clinical applications: There will be an increasing emphasis on translating bioinformatics findings into practical clinical applications to improve human health.
-
Greater collaboration between bioinformaticians, biologists, and clinicians: Effective translation of bioinformatics research requires close collaboration between scientists from diverse disciplines. Future publications will likely reflect this increasing interdisciplinary collaboration.
Conclusion:
Recent empirical reports in bioinformatics reflect the field's rapid evolution, driven by the explosion of biological data and the increasing power of computational methods. While significant challenges remain, particularly in managing and interpreting big data, and addressing ethical considerations related to AI, the future of bioinformatics holds immense promise for advancing our understanding of biological systems and improving human health. The ongoing publication of empirical studies is crucial for documenting progress, identifying limitations, and shaping the future direction of this rapidly evolving field. Continuous critical evaluation of published research is essential for ensuring the integrity and reliability of bioinformatics findings. By addressing the challenges and fostering collaboration, the bioinformatics community can leverage the power of big data and AI to unlock new frontiers in biological discovery.
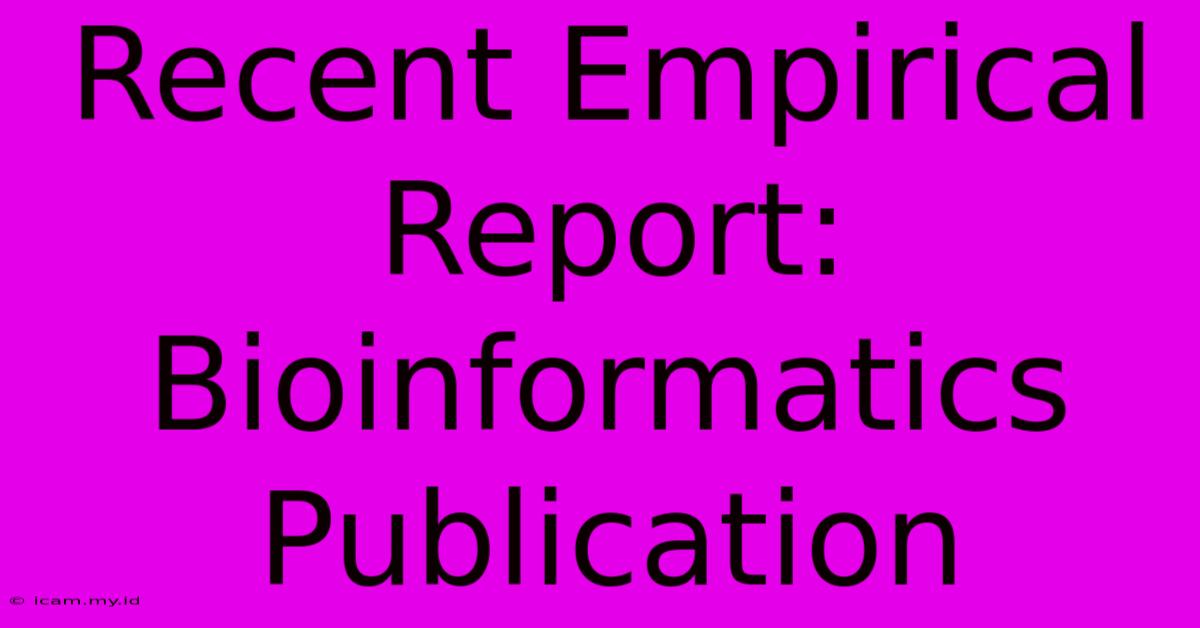
Thank you for visiting our website. Recent Empirical Report: Bioinformatics Publication. We hope the information we provide is helpful to you. Feel free to contact us if you have any questions or need additional assistance. See you next time, and don't forget to save this page!
Kami berterima kasih atas kunjungan Anda untuk melihat lebih jauh. Recent Empirical Report: Bioinformatics Publication. Informasikan kepada kami jika Anda memerlukan bantuan tambahan. Tandai situs ini dan pastikan untuk kembali lagi segera!
Featured Posts
-
Vinicius Jr Out Real Madrids Liverpool Trip
Nov 28, 2024
-
Rare Earth Opportunities And Roadblocks
Nov 28, 2024
-
2031 Rare Earth Metals Market Forecast
Nov 28, 2024
-
Insurance Premium Increase Takafuls Explanation
Nov 28, 2024
-
Trash Pickup Thanksgiving 2024
Nov 28, 2024